Studying satisfaction with life can assist evaluation of the effect of interventions mediating mental disorders, yet frequent or regular monitoring of SWL in longitudinal studies is laborious and often results in high dropout rate. In this study, we applied machine learning to social network data to predict SWL. Based on a variety of feature selection methods and a three-step random forest model, our language-based model for SWL reached an accuracy of 0.58 (SWL split into three classes). Machine-predicted SWL scores showed higher validity coefficients than self-reported SWL scores when predicting certain life outcomes. Using the same method, we built three more models predicting respectively depression, personality, and self-disclosure, the accuracies of which were all over 0.50. Comparison of these models suggest that content words such as negative emotion words are the major indicators of SWL and a multi-step random forest model can make valid predictions for many psychological traits.
HKOSCon 2017LIVE at #HKOSCon2017
Hong Kong Open Source Conference 香港開源年會
Speaker
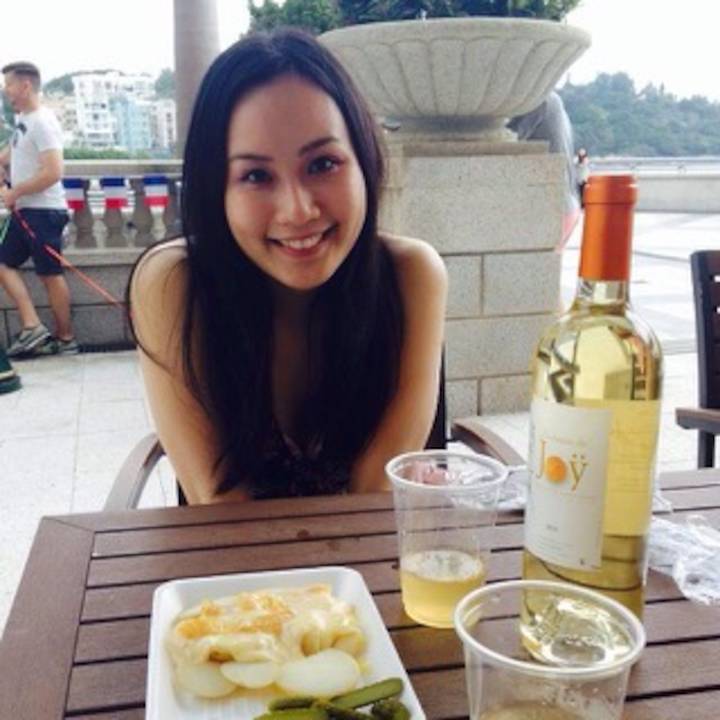
Lushi Chen
I'm a psychologist researches on social media data and user’s psychological traits. During the past two years, I have proactively engaged with social media data and worked towards using a machine learning approach to model user's mental health and psychological traits. Besides using R and Python to conduct data analysis on my studies, I’m also a junior mobile/web app developer with some experience in JavaScript and Meteor Platform.